The International Joint Conference on Artificial Intelligence (IJCAI) has accepted our scientific paper
Back to all articles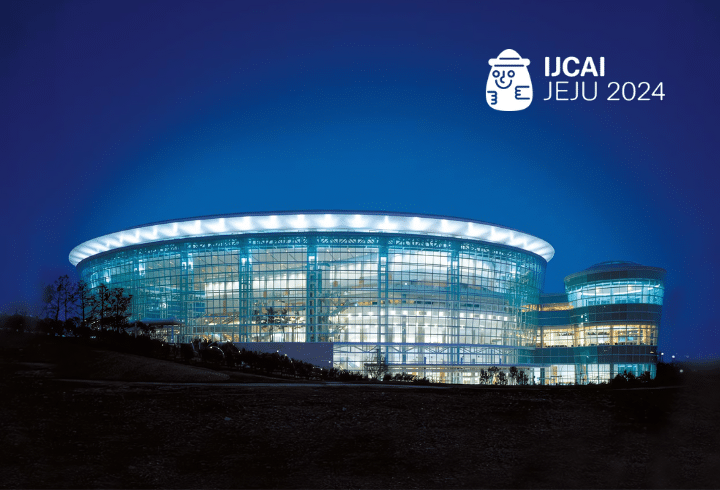
From August 3 to 9, Ekimetrics is in Jeju, Korea, for the IJCAI conference, the premier international gathering of AI researchers.
We’re proud to announce that our scientific paper, “Learning Structural Causal Models through Deep Generative Models: Methods, Guarantees, and Challenges”, has been accepted for presentation at the prestigious IJCAI conference. Carried out by Audrey Poinsot, one of our PhD students supervised by Nicolas Chesneau, our Head of Innovation, Research & Development, this work was developed in collaboration with INRIA and CNRS researchers Alessandro Leite and Michèle Sébag, part of the LISN lab in Paris Saclay University.
The paper provides a comprehensive review of deep structural causal models (DSCMs), focusing on their ability to answer counterfactual queries using observational data within known causal structures. It delves into the characteristics of DSCMs by analyzing the hypotheses, guarantees, and applications inherent to the underlying deep learning components and structural causal models, fostering a finer understanding of their capabilities and limitations in addressing different counterfactual queries. Furthermore, it highlights the challenges and open questions in the field of deep structural causal modeling, setting the stage for researchers to identify future directions and for practitioners to find the most appropriate methods for their needs.
From an ethical standpoint, this work aims to prevent malicious or naive use of these models by being transparent about their limitations and the plausibility of the assumptions. As a result, for sensitive decision-making applications, we recommend validating all the assumptions made with experts in the concerned fields (e.g., insurance, health, finance, HR) before using these methods.
The acceptance of our paper by the International Joint Conference on Artificial Intelligence is a testament to the hard work and expertise of our PhD and Research & Development teams. This paper represents the innovation coming out of our innovation lab, Eki.lab. As a mission-driven company dedicated to responsible AI, we’re committed to advancing scientific research to provide the best AI solutions for our clients.
To read the scientific paper: Learning Structural Causal Models through Deep Generative Models: Methods, Guarantees, and Challenges